|
ACCESS THE FULL ARTICLE
No SPIE Account? Create one
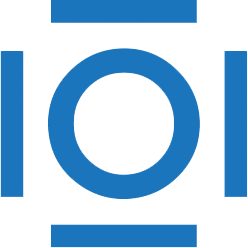
CITATIONS
Cited by 128 scholarly publications.
LIDAR
Earth observing sensors
Landsat
Data modeling
Calibration
Sensors
Vegetation