|
ACCESS THE FULL ARTICLE
No SPIE Account? Create one
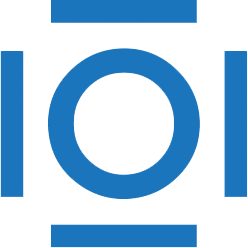
CITATIONS
Cited by 4 scholarly publications.
Image classification
Airborne remote sensing
Remote sensing
Spatial resolution
Image segmentation
Image analysis
Artificial neural networks