|
ACCESS THE FULL ARTICLE
No SPIE Account? Create one
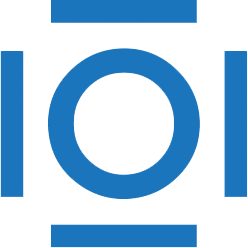
CITATIONS
Cited by 13 scholarly publications.
Image segmentation
Synthetic aperture radar
Feature extraction
Binary data
Image classification
Image filtering
Image processing algorithms and systems