|
ACCESS THE FULL ARTICLE
No SPIE Account? Create one
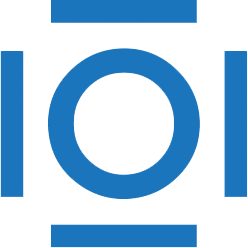
CITATIONS
Cited by 4 scholarly publications.
Detection and tracking algorithms
Target detection
Land mines
Signal to noise ratio
Mathematical morphology
Image filtering
Reconstruction algorithms