|
ACCESS THE FULL ARTICLE
No SPIE Account? Create one
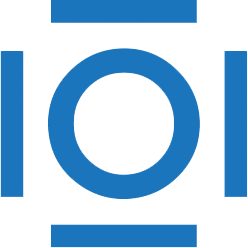
CITATIONS
Cited by 5 scholarly publications.
Image filtering
Synthetic aperture radar
Automatic target recognition
Distortion
Tolerancing
Linear filtering
Detection and tracking algorithms