|
ACCESS THE FULL ARTICLE
No SPIE Account? Create one
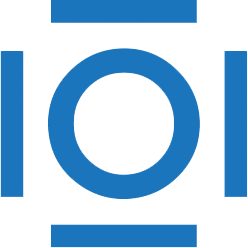
CITATIONS
Cited by 1 scholarly publication.
Neural networks
Reflectivity
Spectral models
Spectroscopy
Data modeling
Data storage
Systems modeling