|
ACCESS THE FULL ARTICLE
No SPIE Account? Create one
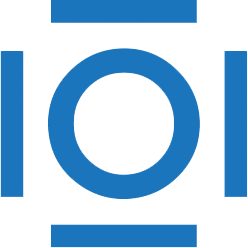
CITATIONS
Cited by 3 scholarly publications.
Image classification
Feature extraction
Binary data
Filtering (signal processing)
Wavelets
Wavelet transforms
Electronic filtering