|
ACCESS THE FULL ARTICLE
No SPIE Account? Create one
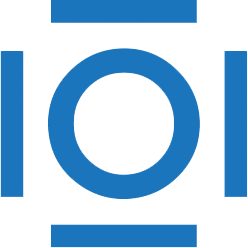
CITATIONS
Cited by 14 scholarly publications and 2 patents.
LIDAR
Signal to noise ratio
Doppler effect
Interference (communication)
Data processing
Profiling
Stochastic processes