|
ACCESS THE FULL ARTICLE
No SPIE Account? Create one
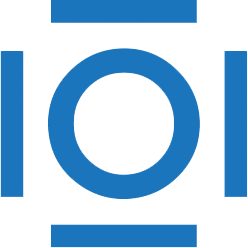
CITATIONS
Cited by 1 scholarly publication.
Data modeling
Earth observing sensors
Landsat
Composites
Remote sensing
Environmental sensing
Geographic information systems