|
ACCESS THE FULL ARTICLE
No SPIE Account? Create one
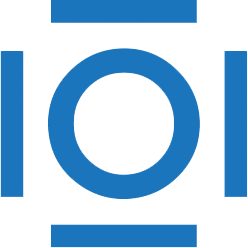
CITATIONS
Cited by 1 scholarly publication.
Data modeling
Expectation maximization algorithms
Hyperspectral imaging
Statistical modeling
Target detection
Hyperspectral target detection
Scanning electron microscopy