|
ACCESS THE FULL ARTICLE
No SPIE Account? Create one
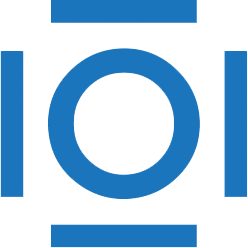
CITATIONS
Cited by 5 scholarly publications.
Target detection
Sensors
Detection and tracking algorithms
Hyperspectral imaging
Algorithm development
Image processing
Optical inspection