|
ACCESS THE FULL ARTICLE
No SPIE Account? Create one
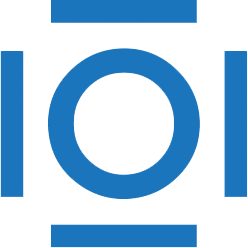
CITATIONS
Cited by 1 scholarly publication.
Image segmentation
Remote sensing
Visualization
Image processing
Algorithm development
Associative arrays
Carbonates