Change detection is an important research direction in the field of remote sensing technology. However, for hyperspectral images, the nonlinear relationship between the two temporal images will increase the difficulty of judging whether the pixel is changed or not. To solve this problem, a hyperspectral change detection method is proposed in which the transformation matrices are obtained by using the constraint formula based on the minimum spectral angle, which uses both spectral and spatial information. Further, a kernel function is used to handle the nonlinear points. There are three main steps in the proposed method: first, the two temporal hyperspectral images are transformed into new dimensional space by a nonlinear function; second, in the dimension of observation, all the observations are combined into a vector, and then the two transformation matrices are obtained by using the formula of spectral angle constraint; and third, each pixel is given weight with a spatial weight map, which combined the spectral information and spatial information. Study results on three data sets indicate that the proposed method performs better than most unsupervised methods. |
ACCESS THE FULL ARTICLE
No SPIE Account? Create one
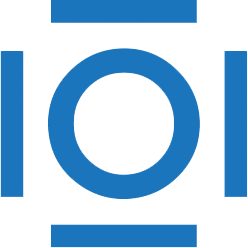
CITATIONS
Cited by 4 scholarly publications.
Hyperspectral imaging
Matrices
Principal component analysis
Signal to noise ratio
Single crystal X-ray diffraction
Remote sensing
Sensors