The generalized bilinear model (GBM) has been one of the most representative models for nonlinear unmixing of hyperspectral images (HSI), which can consider the second-order scattering of photons. Recently, robust GBM-low-rank representation (RGBM-LRR) for nonlinear unmixing of HSI has been introduced to capture the spatial correlation of HSI using LRR with nuclear norm minimization (NNM). However, NNM is used to approximate the matrix rank by shrinkage all singular values equally. The singular values have distinct physical significance in many real applications, and NNM may not be able to estimate the matrix rank accurately. To overcome the above issue, a robust GBM with a weighted low-rank representation (RGBM-WLRR) approach is proposed using weighted nuclear norm minimization, which mitigates the penalty on larger singular values by assigning a small weight, so the corresponding shrinkage is small and also takes serious shrinkage on small singular values by assigning larger weights to them. The proposed model is solved using an iterative alternating direction method of the multipliers. A series of experiments with real datasets and a simulated HSI with varying rank and signal-to-noise ratio reveals that RGBM-WLRR performs significantly better than the state-of-the-art algorithms in terms of signal-to-reconstruction error, root-mean-square error, and spectral angle distance. |
ACCESS THE FULL ARTICLE
No SPIE Account? Create one
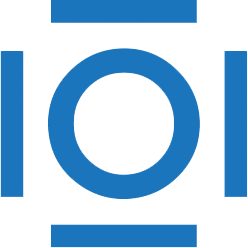
CITATIONS
Cited by 1 scholarly publication.
Signal to noise ratio
Computer simulations
Hyperspectral imaging
Mathematical modeling
Data modeling
Performance modeling
Fluctuations and noise