|
ACCESS THE FULL ARTICLE
No SPIE Account? Create one
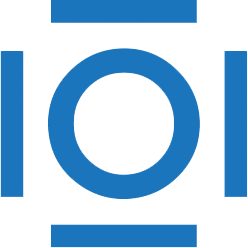
CITATIONS
Cited by 1 scholarly publication.
Error analysis
Data modeling
Image classification
Matrices
Statistical analysis
Fuzzy logic
Performance modeling