Congenital heart failure (CHF) due to congestion in the blood is a serious cardiac problem correlated with crippling symptoms and leading to a rising death rate, monumental health care spending, and reduced quality of life. Heart disease prevention is among the most crucial functions of any medical system, as many people are prone to heart attacks worldwide. Although several segmentation methods for great vessels and the heart have been proposed in the research, they are not successful when applied to the health records of congenital heart disease. In this proposed work, the thickness and fat accumulation of most arteries are measured and analyzed, and then the measurement is synthesized with the corresponding width of the blood vessels of arteries; this data is used for training purposes in the convolutional neural network with one-off cross-validation and regularization. Using the CNN model, a confusion matrix is created and different statistical parameters such as accuracy sensitivity, specificity, precision, and f-score are generated. The final average accuracy was 97%, precision was 98.13%, and F-score was 98.36%. The results indicate that the CNN-based strategy can distinguish healthy hearts from those with prior cardiovascular disease. |
ACCESS THE FULL ARTICLE
No SPIE Account? Create one
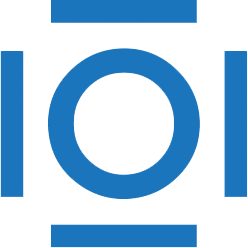
CITATIONS
Cited by 3 scholarly publications.
Heart
Arteries
Data modeling
Performance modeling
Electrocardiography
Feature selection
Blood