To solve the problems of low utilization of spatial features and incomplete contour segmentation in building semantic segmentation of remote sensing images, a building semantic segmentation method in remote sensing images based on dual-path with rich-scale features is proposed. In the shallow spatial path of proposed method, Res2Net module and inception module are used to extract shallow rich scale features to avoid improper use of shallow features affecting segmentation results. In the deep semantic path, ResNet50 combined with hybrid dilated convolution is used as the backbone network, and the obtained high-level semantic features are pooled by a spatial pyramid to capture the deeper multi-scale features. Finally, a new feature fusion module is designed to assign weights to feature maps of different levels extracted from two paths. Experimental results on WHU and Massachusetts building datasets show that the proposed method has higher building extraction accuracy and better generalization ability compared with other semantic segmentation networks. |
ACCESS THE FULL ARTICLE
No SPIE Account? Create one
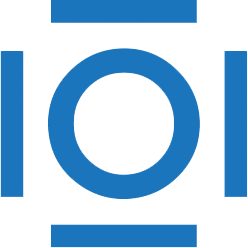
CITATIONS
Cited by 1 scholarly publication.
Buildings
Image segmentation
Remote sensing
Feature extraction
Convolution
Performance modeling
Data modeling