Fine-tuning a pretrained model with real data for a machine learning task requires many hours of manual work, especially for computer vision tasks, where collection and annotation of data can be very time-consuming. We present a framework and methodology for synthetic data collection that is not only efficient in terms of time taken to collect and annotate data, making use of free- and open-source software tools and 3D assets but also beats the state-of-the-art against real data, which is the ultimate test for any similar-to-real approach. We test our approach on a set of image classes from ObjectNet, which is a challenging image classification benchmark test dataset that is designed to be similar in many respects to ImageNet but with a wider variety of viewpoints, rotations, and backgrounds, which can make it more difficult for transfer learning problems. The novelty of our approach stems from the way we create complex backgrounds for 3D models using 2D images laid out as decals in a 3D game engine, where synthetic images are captured programmatically with a large number of systematic variations. We demonstrate that our approach is highly effective, resulting in a deep learning model with a top-1 accuracy of 72% on the ObjectNet data, which is a new state-of-the-art result. In addition, we present an efficient strategy for learning rate tuning that is an order of magnitude faster than regular grid search. |
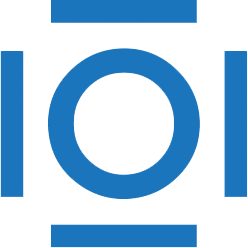
CITATIONS
Cited by 1 scholarly publication.
Lawrencium
3D modeling
Data modeling
Education and training
Machine learning
Light sources and illumination
3D image processing