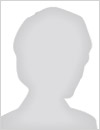
This will count as one of your downloads.
You will have access to both the presentation and article (if available).
Here, we propose an update to the OMI-DB collection system, whereby the storage of all the data is automatically transferred to the cloud on collection. This change in the data collection paradigm reduces the reliance of physical servers at each site; allows greater scope for future expansion; and removes the need for dedicated backups and improves security. Moreover, with the number of applications to access the data increasing rapidly with the maturity of the dataset cloud technology facilities faster sharing of data and better auditing of data access. Such updates, although may sound trivial; require substantial modification to the existing pipeline to ensure data integrity and security compliance. Here, we describe the extensions to the OMI-DB collection pipeline and discuss the relative merits of the new system.
The requirements of remote, collaborative image review sessions, such as those required to run a remote interval cancer review, are variable and demand a flexible and configurable software solution that is not currently available on commercial workstations. The wide range of requirements for both collection and remote review of interval cancers has precipitated the creation of extensible medical image viewers and accompanying systems.
In order to allow remote viewing, an application has been designed to allow workstation-independent, PACS-less viewing and interaction with medical images in a remote, collaborative manner, providing centralised reporting and web-based feedback. A semi-automated process, which allows the centralisation of interval cancer cases, has been developed. This stand-alone, flexible image collection toolkit provides the extremely important function of bespoke, ad-hoc image collection at sites where there is no dedicated hardware.
Web interfaces have been created which allow a national or regional administrator to organise, coordinate and administer interval cancer review sessions and deploy invites to session members to participate. The same interface allows feedback to be analysed and distributed.
The eICR provides a uniform process for classifying interval cancers across the NHSBSP, which facilitates rapid access to a robust 'external' review for patients and their relatives seeking answers about why their cancer was 'missed'.
X-ray attenuation of adipose breast tissue: in-vitro and in-vivo measurements using spectral imaging
Here, we describe an extension to the OMI-DB whereby a range of imaging features and descriptors are pre-calculated using a high throughput approach. The ability to calculate multiple imaging features and data from the acquired images would be valuable and facilitate further research applications investigating detection, prognosis, and classification. The resultant data store contains more than 10 million quantitative features as well as features derived from CAD predictions. Theses data can be used to build predictive models to aid image classification, treatment response assessment as well as to identify prognostic imaging biomarkers.
6825 mammograms from our mammography image database were segmented to find the breast outline. The outlines were averaged and smoothed to produce an average outline for each laterality and radiographic projection.
Lesions in 3304 mammograms with malignant findings were mapped on to a standardised breast image corresponding to the average breast outline using piecewise affine transforms. A four dimensional probability distribution function was found from the lesion locations in the cranio-caudal and medio-lateral radiographic projections for calcification and noncalcification lesions. Lesion locations sampled from this probability distribution function were mapped on to individual mammograms using a piecewise affine transform which transforms the average outline to the outline of the breast in the mammogram.
The four dimensional probability distribution function was validated by comparing it to the two dimensional distributions found by considering each radiographic projection and laterality independently. The correlation of the location of the lesions sampled from the four dimensional probability distribution function across radiographic projections was shown to match the correlation of the locations of the original mapped lesion locations.
The current system has been implemented as a web-service on a server using the Python Django framework. The server performs the sampling, performs the mapping and returns the results in a javascript object notation format.
View contact details
No SPIE Account? Create one