|
ACCESS THE FULL ARTICLE
No SPIE Account? Create one
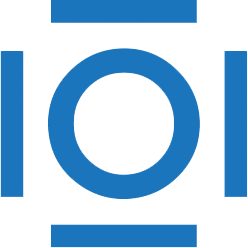
CITATIONS
Cited by 5 scholarly publications.
Image segmentation
Prostate
Magnetic resonance imaging
Binary data
3D image processing
3D modeling
Convolutional neural networks