|
ACCESS THE FULL ARTICLE
No SPIE Account? Create one
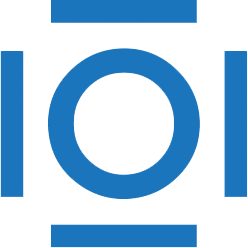
CITATIONS
Cited by 12 scholarly publications.
Databases
Feature extraction
Image retrieval
Medical imaging
Computed tomography
Picture Archiving and Communication System
Neurons