|
ACCESS THE FULL ARTICLE
No SPIE Account? Create one
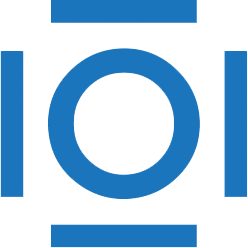
CITATIONS
Cited by 3 scholarly publications.
Data modeling
Genetics
Neuroimaging
Brain
Matrices
Alzheimer's disease
Analytical research