|
ACCESS THE FULL ARTICLE
No SPIE Account? Create one
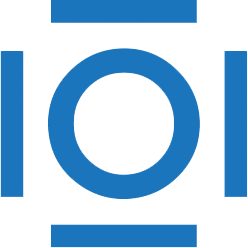
CITATIONS
Cited by 17 scholarly publications and 1 patent.
Image segmentation
Computed tomography
3D image processing
3D modeling
Image processing
Medical imaging
Chest