|
ACCESS THE FULL ARTICLE
No SPIE Account? Create one
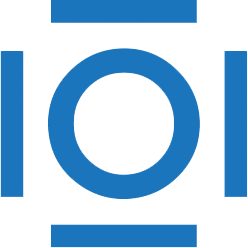
CITATIONS
Cited by 1 scholarly publication.
Heart
Image segmentation
Ultrasonography
Convolutional neural networks
Convolution
Neural networks
Data modeling