|
ACCESS THE FULL ARTICLE
No SPIE Account? Create one
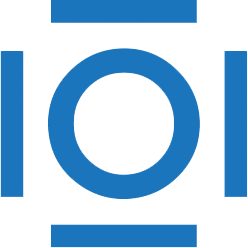
CITATIONS
Cited by 5 scholarly publications.
Data modeling
Hyperspectral imaging
Data centers
Image processing
Data processing
Sensors
Image classification