|
ACCESS THE FULL ARTICLE
No SPIE Account? Create one
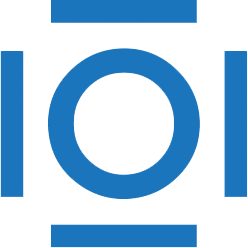
CITATIONS
Cited by 2 scholarly publications.
Sensors
Sensor networks
Neural networks
Detection and tracking algorithms
Defect detection
Environmental sensing
Algorithm development