|
ACCESS THE FULL ARTICLE
No SPIE Account? Create one
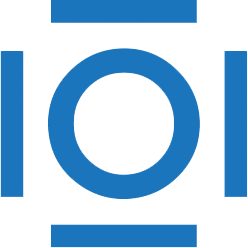
CITATIONS
Cited by 12 scholarly publications.
Image registration
Distance measurement
Lung
Convolution
Machine learning
Image processing
Image segmentation