|
ACCESS THE FULL ARTICLE
No SPIE Account? Create one
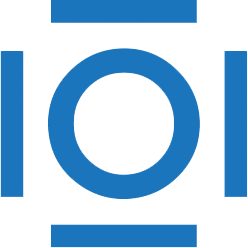
CITATIONS
Cited by 1 scholarly publication.
Computed tomography
Image segmentation
Lung
Image registration
Image analysis
Modeling