|
ACCESS THE FULL ARTICLE
No SPIE Account? Create one
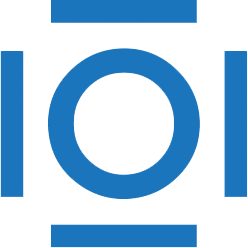
CITATIONS
Cited by 2 scholarly publications.
Breast cancer
Neurons
Data modeling
Tumor growth modeling
Cancer
Pathology
Digital imaging