|
ACCESS THE FULL ARTICLE
No SPIE Account? Create one
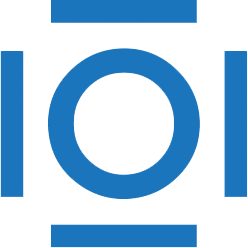
CITATIONS
Cited by 6 scholarly publications and 1 patent.
Principal component analysis
Algorithm development
Matrices
Electroencephalography
Error analysis
Analytical research
Antennas