|
ACCESS THE FULL ARTICLE
No SPIE Account? Create one
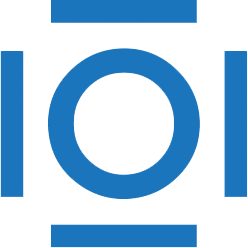
CITATIONS
Cited by 2 scholarly publications.
Image quality
Super resolution
Satellite imaging
Satellites
Medical imaging
Image processing
Neural networks