|
ACCESS THE FULL ARTICLE
No SPIE Account? Create one
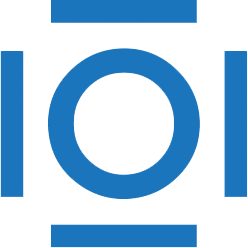
CITATIONS
Cited by 18 scholarly publications.
Systems modeling
Data modeling
Classification systems
Binary data
Feature extraction
Quality systems
Alternate lighting of surfaces