|
ACCESS THE FULL ARTICLE
No SPIE Account? Create one
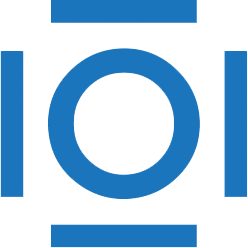
CITATIONS
Cited by 5 scholarly publications.
Surface properties
Image classification
Image processing
Sensors
Optical inspection
Matrices
Detection and tracking algorithms