|
ACCESS THE FULL ARTICLE
No SPIE Account? Create one
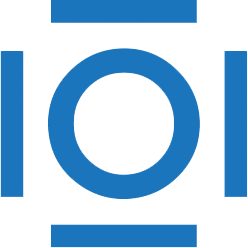
CITATIONS
Cited by 1 scholarly publication.
Data modeling
Synthetic aperture radar
Performance modeling
Target recognition
Convolution
Feature extraction
Neural networks