|
ACCESS THE FULL ARTICLE
No SPIE Account? Create one
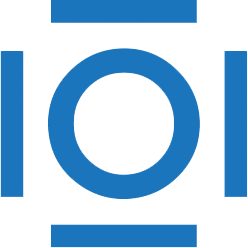
CITATIONS
Cited by 4 scholarly publications.
Agriculture
Machine learning
Analytical research
MODIS
Classification systems
Control systems
Imaging systems