|
ACCESS THE FULL ARTICLE
No SPIE Account? Create one
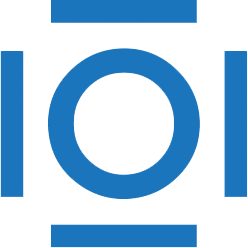
CITATIONS
Cited by 1 scholarly publication.
Video
Databases
Neural networks
Distortion
Image quality
Neurons
Artificial neural networks