|
ACCESS THE FULL ARTICLE
No SPIE Account? Create one
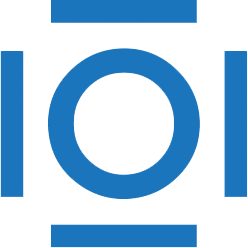
CITATIONS
Cited by 2 scholarly publications.
Expectation maximization algorithms
Lung cancer
Computed tomography
Cancer
Computer aided diagnosis and therapy
Radiology
Artificial intelligence