|
ACCESS THE FULL ARTICLE
No SPIE Account? Create one
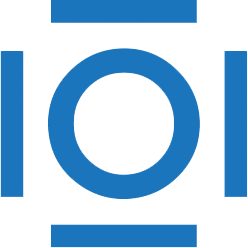
CITATIONS
Cited by 1 scholarly publication.
Tumors
Lung
Image segmentation
Chest
Computed tomography
3D image processing
Lung cancer