|
ACCESS THE FULL ARTICLE
No SPIE Account? Create one
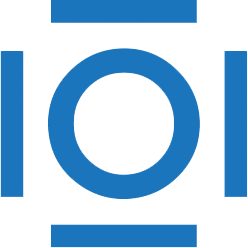
CITATIONS
Cited by 1 scholarly publication.
Convolution
Neural networks
Performance modeling
Convolutional neural networks
Agriculture
Machine learning
Network architectures