|
ACCESS THE FULL ARTICLE
No SPIE Account? Create one
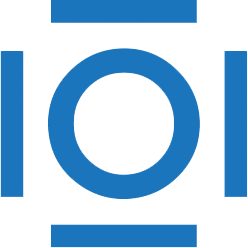
CITATIONS
Cited by 1 scholarly publication.
Neural networks
Hough transforms
Image processing
Convolutional neural networks
Computer science