|
ACCESS THE FULL ARTICLE
No SPIE Account? Create one
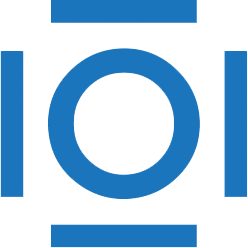
CITATIONS
Cited by 1 scholarly publication.
Feature selection
Distance measurement
Alzheimer's disease
Brain diseases
Brain imaging
Legal
Magnetism