|
ACCESS THE FULL ARTICLE
No SPIE Account? Create one
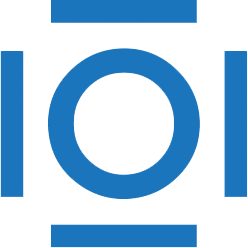
CITATIONS
Cited by 1 scholarly publication.
Control systems
Machine learning
Information fusion
Warfare
Analytics
Computer security
Data fusion