|
ACCESS THE FULL ARTICLE
No SPIE Account? Create one
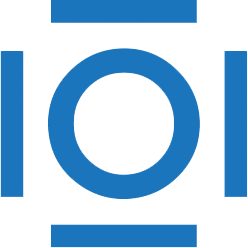
CITATIONS
Cited by 2 scholarly publications.
Robotics
Neural networks
Artificial intelligence
Machine vision
Rule based systems
Visual process modeling
Visualization