|
ACCESS THE FULL ARTICLE
No SPIE Account? Create one
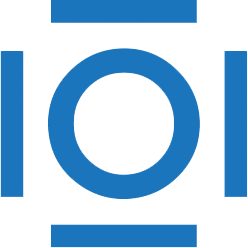
CITATIONS
Cited by 9 scholarly publications.
Machine learning
Matrices
Signal processing
Statistical analysis
Stochastic processes
Image processing
Lithium