|
ACCESS THE FULL ARTICLE
No SPIE Account? Create one
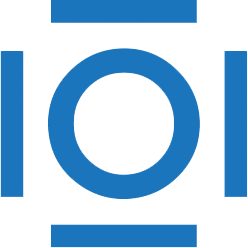
CITATIONS
Cited by 3 scholarly publications.
Neural networks
Artificial neural networks
Feature extraction
Signal detection
Signal processing
Interference (communication)
Acoustics