|
ACCESS THE FULL ARTICLE
No SPIE Account? Create one
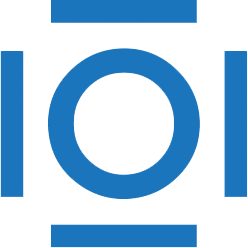
CITATIONS
Cited by 2 scholarly publications.
Neural networks
Reconstruction algorithms
Binary data
Image analysis
Image restoration
Imaging systems
Network architectures