|
ACCESS THE FULL ARTICLE
No SPIE Account? Create one
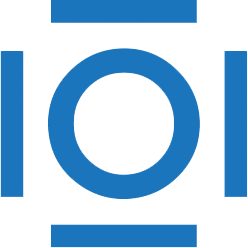
CITATIONS
Cited by 1 scholarly publication.
Genetic algorithms
Data modeling
Stochastic processes
Genetics
Magnetorheological finishing
Image processing
Statistical analysis