|
ACCESS THE FULL ARTICLE
No SPIE Account? Create one
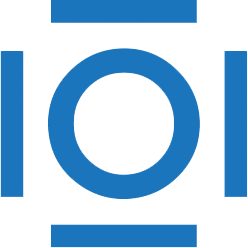
CITATIONS
Cited by 2 scholarly publications.
Nonlinear control
Neural networks
Binary data
Control systems
Neurons
Evolutionary algorithms
Genetic algorithms