|
ACCESS THE FULL ARTICLE
No SPIE Account? Create one
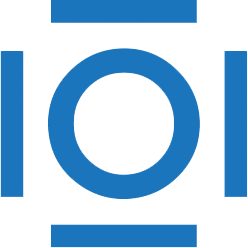
CITATIONS
Cited by 5 scholarly publications.
Complex systems
Signal to noise ratio
Artificial neural networks
Modeling
Network architectures
Neural networks