|
ACCESS THE FULL ARTICLE
No SPIE Account? Create one
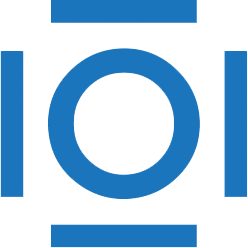
CITATIONS
Cited by 1 scholarly publication.
Fractal analysis
Image segmentation
Mathematical modeling
Visualization
Natural surfaces
Statistical modeling
Lithium